Quantitative Nucleic Acid Test vs. Antigen Testing: Which One to Choose for COVID-19?
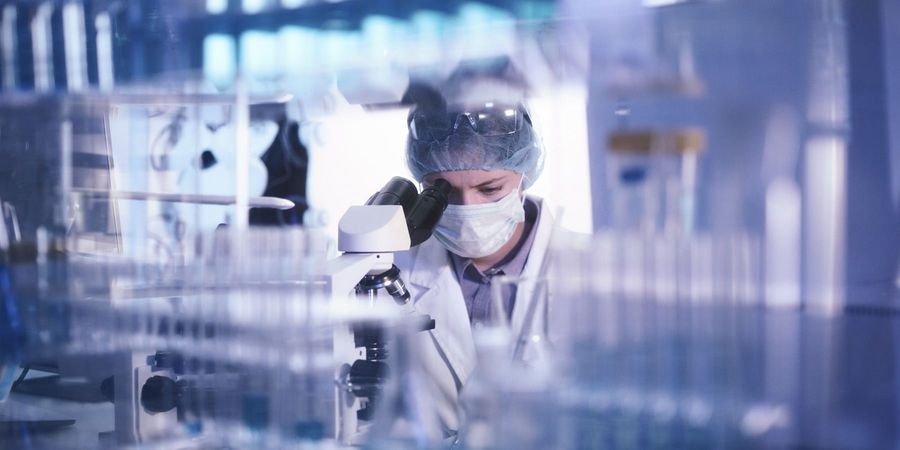
In the fight against the COVID-19 pandemic, testing has been a critical tool for identifying infected persons and controlling the spread of the virus. However, there are different types of tests for detecting the presence of the SARS-CoV-2 virus, each with its advantages and limitations. Two common types of tests are nucleic acid amplification tests (NAATs) and antigen tests.
NAATs, such as RT-PCR, are the gold standard for COVID-19 testing, as they can detect very small amounts of the viral genetic material, even in asymptomatic persons. However, NAATs are expensive, time-consuming, and require laboratory infrastructure and skilled personnel. Also, the results may take hours to days to obtain, delaying contact tracing and isolation measures.
Antigen tests, on the other hand, are cheaper, faster, and more convenient to use than NAATs. Antigen tests detect protein fragments of the virus in nasal or throat swabs and can provide results in minutes. However, antigen tests are less sensitive than NAATs, especially in the early stages of infection or in asymptomatic cases, and may miss some infected persons who are actually contagious. Also, antigen tests may produce more false-positive results, leading to unnecessary isolation and testing.
Given these trade-offs, public health organizations have recommended different strategies for utilizing NAATs and antigen tests, depending on the local epidemiological situation, test availability, and programmatic priorities. Six testing algorithms have been proposed, and a recent study published in BMC Public Health aimed to compare these strategies quantitatively based on their expected performance.
The study utilized a decision analysis approach to simulate the expected outcomes of each testing algorithm in a population of 100,000 persons seeking testing, assuming a prevalence of 10% (which is higher than the current global average). The primary outcomes assessed were the number of missed cases, the number of false-positive diagnoses, and the total test volumes (including both NAATs and antigen tests).
The six testing algorithms were as follows:
1. NAAT only: all persons are tested with NAATs, regardless of symptoms or exposure history.
2. Symptom-based NAAT: only persons with COVID-19 symptoms or known exposure are tested with NAATs; others are tested with antigen tests.
3. Pre-test probability NAAT: persons with a high pre-test probability (e.g., close contacts of confirmed cases) are tested with NAATs initially; others are tested with antigen tests.
4. Confirmatory NAAT: all persons are tested with antigen tests; those with negative results but with symptoms or exposure history are retested with NAATs.
5. Protocol-based NAAT: a fixed ratio (e.g., 1:10) of persons are tested with NAATs; the rest are tested with antigen tests.
6. Symptom-based confirmatory NAAT: only symptomatic persons are tested with antigen tests; those with negative results but with symptoms or exposure history are retested with NAATs.
The simulation results showed that no single testing algorithm was optimal across all settings and programmatic priorities. However, some algorithms performed better than others in specific outcomes.
For example, algorithms that used NAATs to confirm all negative antigen results had the lowest number of missed cases but required the highest NAAT capacity (92,200 tests, in addition to 100,000 antigen tests, at 10% prevalence). This strategy may be suitable for outbreak investigation or settings with high risk of transmission, but it may not be sustainable in resource-limited contexts.
Selective use of NAATs to confirm antigen results when discordant with symptom status (e.g., symptomatic persons with negative antigen results) resulted in the most efficient use of NAATs, with only 25 NAATs needed to detect one additional case compared to exclusive use of antigen tests. This strategy may be more feasible in settings with medium to high prevalence and limited NAAT capacity.
Other strategies, such as symptom-based NAAT and protocol-based NAAT, were less effective in reducing missed cases but had advantages in reducing test volumes and resource use. For example, protocol-based NAAT required only 10,110 NAATs and 100,000 antigen tests to achieve a similar number of missed cases compared to confirmatory NAAT (with 35,320 NAATs and 100,000 antigen tests). This strategy may be useful in settings with low prevalence and limited resources.
Overall, the study highlights the importance of adopting a context-specific approach to COVID-19 testing, considering factors such as test capacity, test sensitivity, programmatic priorities, and resource constraints. No single testing algorithm can address all these factors optimally, and a trade-off between them is inevitable. Therefore, public health organizations should be transparent about the assumptions, limitations, and uncertainties of their testing strategies and regularly update them based on new evidence and feedback from stakeholders.
In conclusion, while both NAATs and antigen tests have their advantages and limitations for detecting SARS-CoV-2, the choice of testing strategy should be based on a rational and evidence-informed assessment of the local situation and needs. Quantitative decision analysis, such as the one presented in this study, can provide a useful framework for comparing different testing algorithms and guiding policy decisions. However, the ultimate goal of COVID-19 testing is not just to detect cases but also to prevent transmission and save lives, and this requires a comprehensive and integrated approach to public health measures.